What are the Ethical Issues in Data Science Applications?
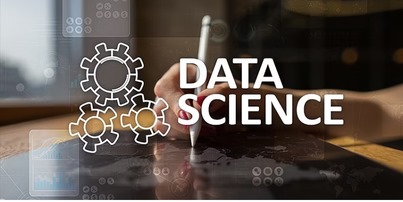
Due to the fast adoption of advanced technology in data processing, ethical factors in data science are compulsory. Since data drives organization decision making functions, elements like privacy, bias and responsibility have to be checked to make sure organizations fully utilize data rightfully. It is imperative to comprehend all of these ethical dilemmas in order to promote sound credibility and accountability of data science uses. Joining the Data Science Course in Chennai will enhance your understanding of Machine Learning Models.
Privacy Concerns in Data Collection
A number of data science applications require data collection, which always raises a number of questions concerning ethicality, especially about privacy. With the increased use of information technology in organizations, the amount of personal data collected from different sources, such as social media sites, online purchases, and mobile devices, exposes their privacy. Privacy concerns involve circumstances where data was gathered without the knowledge of the user or circumstances where the data was gathered from users with no regard for how it will be utilized. Another important principle; organisations must be clear about the process of data collection and individuals must have the ability to control their data. Failure to consider these issues is an issue of privacy that might make potential users have low levels of trust and it might also be in violation of the law.
Bias in Data and Algorithms
Bias in data science is another pressing ethical issue. In this case, observations or numbers are gathered from information that is obtained from past records and databases that contain prejudices of society. This is because when such biased datasets are used to train the different algorithms, the formed models will have a way of exacerbating such biases. For example, beginning with assigning crime statistics that are skewed to one race, a predictive policing algorithm can single out residents of a particular race as criminals, while a hiring algorithm can exclude candidates of a certain color by using prejudiced job data. Bias solutions, therefore, increase the usage of diversity datasets and the usage of fairness measurements that determine the effects of algorithms. Data scientists should also deliberately pursue ways by which biases can be eliminated or reduced when developing applications.
Accountability and Responsibility
It then highlights how accountability is generally a multifaceted area to address in data science when thinking about ethical concerns, especially decision-making. When algorithmic decisions are made by computer programs across different sectors, including but not limited to healthcare, finance and the justice system, there arises a rightful concern of who is to blame when decisions by these algorithms go wrong. In the mismatch where an algorithm provides a wrong recommendation, resulting in adverse outcomes, deciding who is at fault becomes very difficult. This means that the data scientists, organizations and stakeholders involved need to get their side of the story right and ensure that there is a possibility of remedy when harm occurs. This involves creating ethical frameworks and governance structures to guide the responsible use of data science technologies.
Data Security and Protection
Information preservation remains one of the critical objectives in the ethical use of data in data science. In a world where every day a new cyberattack or data leakage is reported, the protection of such data becomes critical. The ethical problem comes when organizations design their systems in such a way that they are vulnerable to such criticisms and consequent illegitimate access or use. Hence, it becomes incumbent on data scientists and organizations in general to do all they can to prevent attacks. Besides, it is necessary to be open about practices that are being taken to protect user data and possible threats to it as well. The use of user data should be governed by the ethical imperative of Data Protection lifecycle management.
Ethical Use of Artificial Intelligence
The integration of artificial intelligence (AI) in data science applications raises unique ethical considerations. AI systems often operate autonomously, making decisions based on data-driven insights. But when such decisions have profound repercussions on people or groups, using scripts raises ethical issues, as the systems take decisions autonomously. These points concerning algorithmic transparency, the possibility of ‘explainability’ of the result and even the possibility of discrimination should be taken into consideration. Nowadays, data scientists should take the gloves and make sure that AI systems are created with the specific ideas of ethically in mind, including fairness, accountability and transparency. This means that there is a need to have ethical principles and standards that check all AI development so as to avoid high-risk implications of AI implementation.
Ethical Implications of Predictive Analytics
An interesting subset of data science applications that raises ethical concerns relates to its use in decision-making: predictive analytics. However, even when accurate predictive models are developed the intrinsic nature of such systems turns them into vehicles of extrapolation of the existing biases and prejudices with the risk of deepening social inequality. For instance, recommendation services may employ an algorithm that gives some specific populations a higher priority than others in areas such as health. There are some ethical practices which should be followed when engaging in predictive analytics to avoid developing and applying wrong models that hurt society. The application of model-driven decisions must be critically analyzed by data scientists to achieve more positive results for all entities involved.
Environmental Impact of Data Science
An important ethical consideration that has remained opaque is the environmental effect of data science solutions. The quantity of energy generated when large quantities of data are collected, stored, and analyzed has potentially large environmental impacts. This imposed a high energy demand on the facility needed to house the servers, and this, in turn, causes carbon emissions and climate change. Ethical business concerns should also include the sustainability of data science processes instead of calling on organisations to take stock of their impacts on the environment. Using energy-efficient technologies, seeking the most efficient algorithms with the least resource utilization, and general adherence to principles of sustainability may also be observed to make the use of data science for ethical purposes with respect to the environment. Enroll in the Data Science Courses in Bangalore, which provide a deeper understanding of Data Science concepts.
It underlines the importance of discussing the ethical questions arising from the data science applications so as to make innovation more trustworthy. We believe that through creating dedication on openness, justice, and responsibility, the potential contributions of Fig based analytics can be maximized without compromising civil liberties and social values.