Challenges and Use Cases of Robotic Process Automation in Healthcare
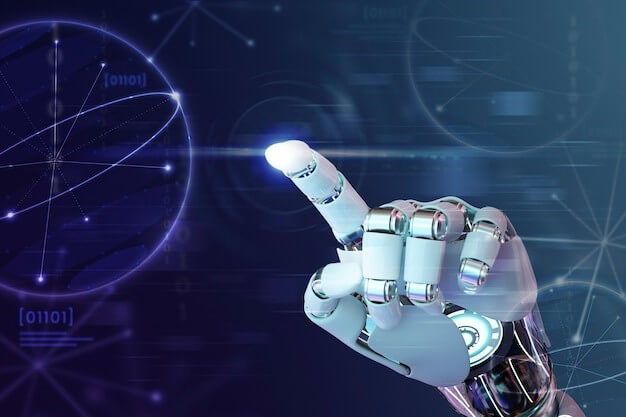
The manual execution of healthcare operations significantly undermines efficiency and severely compromises the quality of the patient experience. Given that healthcare enterprises operate in real-time environments, precision and promptness are pivotal factors contributing to overall efficiency. Furthermore, the well-being of both staff members and patients holds significant importance in this context. Consequently, the manual handling of healthcare operations results in increased healthcare operational expenses and prolonged turnaround times for patient care delivery. The integration of robotic process automation in healthcare operations offers the ability to virtually automate a wide array of manual and repetitive tasks, thereby diminishing the reliance on human intellect. Consequently, this technological advancement can yield notable benefits such as heightened operational efficiencies, cost reductions, error mitigation, empowerment of staff, and an overall enhancement of patient care and experiences.
Challenges in Implementing Robotic Process Automation in Healthcare Faced by Healthcare Organizations
1. Cybersecurity Concerns
Healthcare organizations grapple with the formidable challenge of safeguarding sensitive patient data from cyber threats. In the US market, more than 13 million healthcare or patient records are pilfered and traded annually, often involving IT incidents that implicate individuals within the security chain. Consequently, preserving data privacy and confidentiality stands as the foremost concern for any healthcare institution.
2. Handling Vast Data Volumes
The manual decoding and processing of data or the utilization of various tools for this purpose prove to be arduous and time-intensive tasks. Additionally, the complexities associated with storing patient data in legacy systems and ensuring appropriate access for healthcare professionals further compound the challenge.
3. Controlling Rising Costs
Healthcare providers face the daunting challenge of reducing the cost of healthcare delivery while simultaneously enhancing its quality and increasing revenues. This is particularly challenging in an industry that operates in real-time, leaving little room for resource optimization.
4. Workforce Volatility
The United States is projected to face a significant shortage of more than 3.2 million lower-wage healthcare workers, including medical assistants, home health aides, and nursing assistants, within the next five years. This impending workforce gap threatens to diminish the quality of healthcare services offered nationwide.
Use Cases of Robotic Process Automation in Healthcare
-
Patient Eligibility & Registration
Robotic process automation in healthcare streamlines the patient registration process by implementing RPA bots, which can accelerate every aspect, including account setup, history verification, element processing, and billing management.
-
Insurance Eligibility Verification
Eliminate the repetitive task of manually inputting patient information from insurance cards for eligibility checks. Instead, automate data extraction and processing to verify the pertinent insurance plan details and update them on the Electronic Health Record (EHR) portal.
-
Patient Scheduling
RPA bots can be programmed to efficiently schedule patient appointments based on predefined workflows that consider factors like doctor availability, location, and diagnosis with robotic process automation in healthcare. Additionally, these bots offer versatile multi-channel support.
-
Electronic Health Record
Replace manual management of health records with RPA bots that can continuously monitor records from various sources, retrieve PDFs, and extract medical information from diverse documents using Optical Character Recognition (OCR) technology.
-
Admission and Discharge Processes
Robotic process automation in healthcare enhances the efficiency of admission and discharge procedures by utilizing RPA bots to collect data, identify missing tests, flag high-priority discharge cases, send reminders, generate appointment alerts, and dispatch follow-up reminders.
Process of Data Mining
For optimal effectiveness, data analysts typically adhere to a specific sequence of tasks throughout the data mining process. This structured approach helps prevent potential issues that may arise during analysis, which could have been preemptively addressed. The process of data mining is typically divided into the following stages.
Step 1: Understanding the Business Context
Before diving into data handling and analysis, it’s essential to grasp the organization’s objectives in data mining. Understand the company’s goals, current business situation, and insights from a SWOT analysis. This initial step sets the criteria for success in the process of data mining.
Step 2: Explore the Data Landscape
Once the business problem is clear, shift your focus to data considerations. Identify data sources, security measures, storage methods, data collection strategies, and the expected analysis outcomes. Assess data limitations and how they impact the mining process.
Step 3: Data Preparation
Gather, upload, extract, or generate data. Then, clean, standardize, and scrub it for outliers and errors. Evaluate data size to avoid unnecessary computational delays.
Step 4: Model Development
With a clean dataset, commence analysis. Employ various data mining techniques to uncover relationships, trends, associations, or sequential patterns. Consider predictive models to anticipate future outcomes based on past data.
Step 5: Results Assessment
Conclude the data-centric phase by evaluating model findings. Aggregate, interpret, and present results to decision-makers who may not have been involved in the earlier stages. Decisions can then be based on these findings.
Step 6: Implementation and Monitoring
The process of data mining culminates with management taking action based on analysis results. Decisions may lead to strategic adjustments or maintenance of the status quo. Management reviews the business impact and identifies new problems or opportunities, initiating future data mining cycles.
The integration of robotic process automation in healthcare industry addresses critical challenges and offers substantial benefits, including improved operational efficiency, cost reduction, error mitigation, staff empowerment, and enhanced patient care. However, healthcare organizations must contend with cybersecurity concerns, vast data volumes, rising costs, and workforce shortages. When selecting an RPA provider, key considerations include user-friendliness, compliance, versatility, and support. Additionally, the process of data mining follows a structured sequence, beginning with understanding business objectives, exploring data, data preparation, model development, results assessment, and implementation with ongoing monitoring. RPA and data mining together have the potential to revolutionize the healthcare industry.